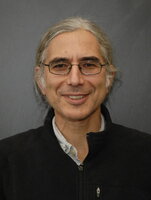
Graduate Courses
The Department of Mathematics at the University of Illinois has historically had a strong reputation in probability, both through its faculty and through the many postdoctoral visitors who have been here. Below is an outline of the general areas of probability theory studied here at Illinois and describes the advanced undergraduate and graduate courses that are offered regularly.
Graduate Study in Probability Theory
Probability theory provides the mathematical framework for the study of experiments for which the outcome is unpredictable by virtue of some intrinsic chance mechanism. The ideas and methods that are continually being developed for this provide powerful tools for many other things, for example, the discovery and proof of new theorems in other parts of mathematics.
Topics of interest to the faculty at the University of Illinois include martingale theory, interacting particle systems, general theory of Markov processes, random fields, stochastic differential equations, diffusion processes, and limit theorems. A common element of these topics is a triple (w, £, P) consisting of a collection of outcomes w, a class £ of subsets A of which are called events, and a probability function P which assigns to each event A a probability P(A). Each of the topics comes about by specifying a collection (X(t): t in T) of functions, called random variables or vectors, defined on 2 taking on values in some prescribed space and probabilistic statements relating the X.
Martingale Theory
In the particular case that the parameter set T is ordered and each real-valued random variable X(t) is related to X(s) for s < t by an averaging property, the process is called a martingale. Martingales were introduced in the late 1930s and extensively developed in the 194Os and 1950s by Emeritus Professor Joseph L. Doob of the University of Illinois. Martingale theory has turned out to be a powerful tool for the modern probabilist. Not only are martingales of interest in their own right as models for gambling systems but they occur naturally in many parts of analysis. Applications range from studying solutions of partial differential equations to studying geometrical properties of Banach spaces.
Interacting Particle Systems
The topic of interacting particle systems has its origin in statistical mechanics of physics and became a branch of probability theory in the late 1960s with the pioneer work of R. L. Dobrushin of the Soviet Union and F. Spitzer of Cornell University. The objective of this topic is to describe the random time evolution of interacting particle systems and to analyze the long-term probability characteristics of the system. One goal in particular is to explain the phenomenon of phase transition of physical systems.
By taking the parameter set T to be the positive reals and specifying that the values of the X(t) are vectors with countably many components, each of which describes the dynamic behavior of a single particle, an interacting particle system is obtained if the probabilistic statements relating the X(t) bring in interactions between components. The tools used in studying such systems involve a blend of classical probability, functional analysis, and martingale theory. An important technique in this topic involves the use of a coupling by which a particle system is compared with a system about which something is known in order to gain some insight into the original system.
Interacting particle systems have been used as models for magnetism, spread of infections, tumor growth, and behavior systems.
Markov Processes
Markov processes have a long history with the first significant results being obtained by the Soviet mathematician A. A. Markov in 1906. Such processes (X(t): t in T) require that T be a subset of the reals and have the property that probability statements concerning a future value given the history of the process up to the present depends only upon the value at the present time.
Using the fundamental solution of the heat equation in n-dimensions to specify the probability statements relating the X(t), a process (X(t): t ³ 0) is obtained which is called n-dimensional Brownian motion. Noted scientists like A. Einstein and N. Weiner have made important contributions to the study of Brownian motion. A fundamental connection between Brownian motion and analysis was made in the 1940s by S. Kakutani. J. L. Doob expanded upon and exploited this connection in the 1950s to prove new theorems in analysis using probability methods and new theorems in probability theory using known results in analysis. This interplay between probability theory and analysis has been and will continue to be a fertile area of research.
Random Fields
Random fields (X(t): t in T) are obtained by allowing the parameter set T to be multidimensional; for example, by letting T be a subset of n-dimensional Euclidean space. Such a field might come about as a random noise superimposed upon a two-dimensional picture or as the longterm equilibrium distribution of an interacting particle system.
One area of current research on random fields has to do with missing values in the following way. Suppose S is contained in T and the X(r) are assumed to be known for all r in T \ S. We are required to estimate the X(t) for t in S using the given X(r) r in T \ S. Another area has to do with the concept of a Markov random field which can be described crudely and inadequately as follows. Thinking of the (X(r): r in S) as in the "past," the (X(t): t in T \ S) as in the "future," and the (X(s): s in boundary S) as the "present," we require that the past and the future be independent given the present. This topic in probability theory is of recent origin and has the potential for being of great importance in applications.
Stochastic Differential Equations
The study of stochastic differential equations is another active area for probabilists. Such equations were first introduced as a means of constructing probability models for diffusion processes with given diffusion rates and drift rates. The first important work in this area was done in the 1940s by K. Ito. If (b(t): t ³ 0) denotes one-dimensional Brownian motion, the differential dbt cannot be defined in the usual way as in calculus but a meaning can be assigned to it and a calculus of such differentials can be developed. Given smooth functions f and g on the reals, it is then possible to consider the stochastic differential equation dX(T) = f(X(T))db(T) + g(X(T))dt and construct a process (X(T): t ³ 0) which solves the equation. Construction of diffusion processes in this way has become an important tool in physics, engineering, and biological sciences.
Limit Theorems
The first limit theorems go back to de Moivre, Laplace, and the Bernoulli brothers. These theorems state that, under suitable conditions, sums of independent random variables, suitably standardized, have distribution functions which converge to the normal distribution function. During the first part of this century, major new theorems of this kind were proved by Bernstein, Lindeberg, Levy, Feller, and Kolmogorov, among others. The independence assumption was replaced a little later by weaker conditions. The term "limit theorem" has come to describe a variety of results about limiting distributions of sums of random variables, the oscillatory behavior of sample paths of processes, and dependence of limiting results on just a few parameters.
Courses in Probability Theory
The regularly scheduled courses of interest to probability students are Math 461, 466, 561, 562, and 564. Detailed course descriptions are below. Once past these courses, the student is brought up to professional research level through reading courses and special topics courses. At least one topics course is offered each semester by a faculty member who is an expert in that topic.
Math 561: Theory of Probability, I
This is the first half of the basic graduate course in measure-theoretic probability theory. It is offered in the Spring semester of every year. The goal of this course is a fairly rigorous understanding of the basic theory of modern probability. The material in this course is fundamental not only in abstract probabilistic analysis, but also in a various applied areas such as communications theory, queuing theory, and mathematical finance. The materials covered in this course include the following: (1) Basic concepts of probability theory: random variables, distributions, expectations, variances, independence and convergence of random variables; (2) the basic limit theorems: the law of large numbers, large deviations and the central limit theorem; (3) conditional expectations, martingales and applications; (4) Brownian motion, weak convergences of probability measures and construction of the Wiener measure. Sometimes the theory of Markov chains and stationary processes are also covered in the course.
Two of the most recently used textbooks for this course are:
- Probability: Theory and Examples, 2nd Edition, R. Durrett, Duxbury Press, 1996.
- Probability Theory, S. R. S. Varadhan, American Mathematical Society, 2001
The prerequisite for this course is the materials of Math 540.
Math 562: Theory of Probability, II
This is the second half of the basic graduate course in measure-theoretic probability theory. It is offered in the Fall semester of every year. The goal of this course is a good understanding of the theory of Brownian motion and stochastic analysis. The material in this course is fundamental not only in abstract probabilistic analysis, but also in various applied areas such as communications theory, queuing theory, mathematical finance and mathematical physics. The materials covered in this course include the following: (1) Brownian motion and continuous time martingales; (2) stochastic integrals; (3) Ito's formula; (4) Girsanov transforms; (5) stochastic differential equations and martingales problems; (4) diffusion processes. Sometimes applications to other areas such as mathematical finance are also covered in this course.
Two of the most recently used textbooks for this course are:
- Brownian Motion and Stochastic Calculus, 2nd Edition, Karatzas and Shreve, Springer, 1994.
- Stochastic Differential Equations, 5th Edition, B. Oksendal, Springer, 1998.
The prerequisite for this course is the materials of Math 561.
Math 564: Applied Stochastic Processes
This is a graduate course on applied stochastic processes and measure theory is not a prerequisite for this course. It is offered in the Fall semester of every year. The goal of this course is a good understanding of the basic stochastic processes and their applications. This course is designed for those graduate students who are going to need to use stochastic processes in their research but do not have the measure-theoretic background to take the Math 451--Math 452 sequence. The materials covered in this course include the following: (1) discrete time Markov chains; (2) continuous time Markov chains; (3) discrete time martingales, (4) stationary processes; (5) applications to queuing theory and other applied fields. The applications covered in this course can be tailored to the interests of the audience.
Two of the most recently used textbooks for this course are:
- Markov Chains, J. R. Norris, Cambridge University Press, 1997.
- Stochastic Processes, S. M. Ross, Wiley, 1996.
- The prerequisite for this course is the materials of Math 461.
Math 461: Probability Theory I
This is an undergraduate course on basic probability theory. Multiple sections of this course are offered each semester. The materials of this course are essential for various fields. The goal of this course is a good understanding of the basic concepts of probability theory. The materials covered in this course include the following: (1) Axioms of probability; (2) sample spaces with equally likely outcomes; (3) conditional probability and independence; (4) random variables, distribution functions and density functions; (5) expectation and variance of random variables; (6) joint distribution and joint density of random variables, independent random variables; (7) law of large numbers and central limit theorem; (8) moment generating functions and characteristic functions.
The prerequisite for this course is the materials in the undergraduate calculus sequence.
Math 466: Probability Theory II
This is an undergraduate course on applied stochastic processes. It has offered irregularly over the years. The goal of this course is a good understanding of the basic stochastic processes and their applications. This course is designed for those undergraduate students who want to learn more about probability and stochastic processes beyond the materials of Math 361.The materials covered in this course include the following: (1) random walks and discrete time Markov chains; (2) continuous time Markov chains; (3) discrete time martingales; (4) applications.
The prerequisite for this course is the materials of Math 461 and the undergraduate calculus sequence.